Climate change leads to rising sea levels, but lack of precipitation can also lead to the reduction of water levels in rivers. This is the case in the Paraná River in Argentina, and the reduced water level limits how heavy cargo the ships that sail on the river can carry, which can have huge consequences for the shipping industry. Thus, it is important to be able to predict the water level in shallow rivers like Paraná to reduce the risk of under-or over-estimating how much cargo can be carried. This can be done using precipitation forecasts, and it is exactly what two master students from the Norwegian School of Economics (NHH) did, in collaboration with the shipping company Western Bulk.
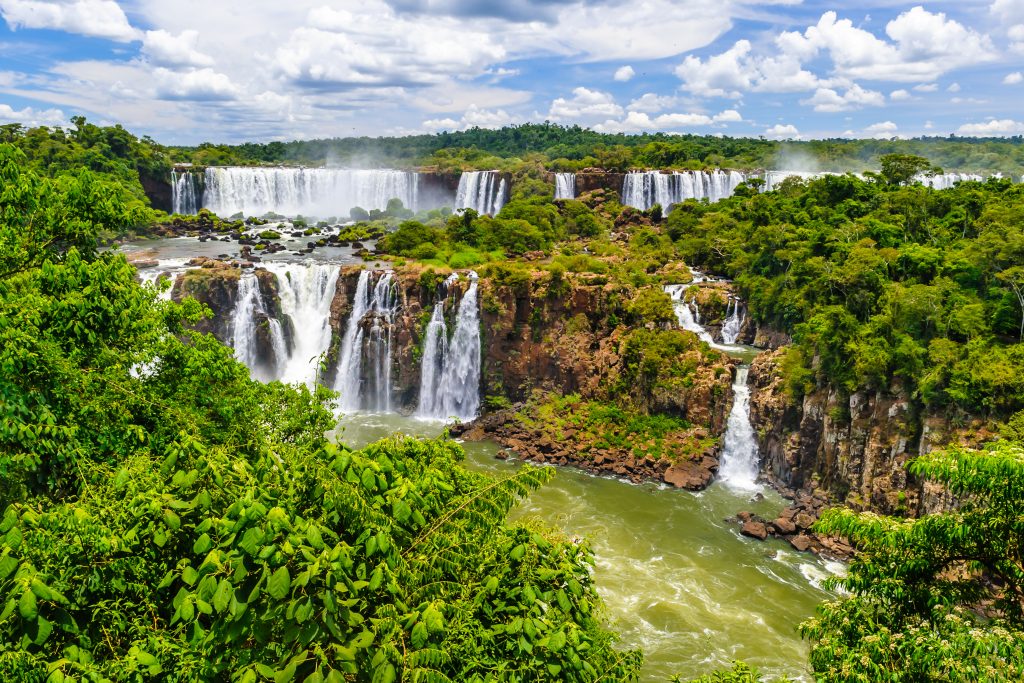
Why estimate river water levels?
Climate change creates more uncertainty in the shipping business. When extreme weather events become more frequent, it becomes more difficult to use historical weather data to predict water levels. Extreme drought, as they have experienced in Argentina, affects whether or not you can go through with the planned cargo. If the river is shallower than the company has calculated, they have to load less grain than planned, which in turn leads to less profit. For example, if Western Bulk estimates that the river is 0,25 metres deeper than it actually is, they can lose a total of 108 375 dollars on this.
It is therefore useful for companies like Western Bulk to have access to tools that can predict water levels 2-12 weeks ahead when the transportation agreement is made. If they can predict the water level in the river, they reduce the risk of miscalculating the cargo, thus improving the company’s bottom line. This can be done by using long-term forecasts for precipitation and surface runoff and investigating the effect these variables have on the water level, and consequently how much cargo a ship can carry.
About the master’s thesis
The master’s students Hanne Utheim and Kaja Henie at NHH have in their thesis investigated if it can be profitable for Western Bulk to use a data source called AIS data to predict the water levels in the Paraná River in Argentina. AIS stands for Automatic Identification System and is a big, open data source that contains, among other things, information about the draft on boats, i.e., the vertical distance between the waterline and the bottom of the hull. It decides how shallow rivers the ship can safely navigate and can therefore also say something about how much cargo the ship can carry. This thesis tries to make a predictive model for the maximum allowable draft for vessels that are sailing through the Paraná River using historical precipitation observations and future precipitation forecasts.
Most ships have AIS installed on the boat that gives an overview of what routes the ship has taken, what speed has been used, and how deep the hull lies under the surface. Some of the data is put in automatically, while some, like the depth of the hull, must be put in manually. In other words, AIS contains large amounts of data that is regularly updated and offers an opportunity to optimise business decisions based on historical data.
Today the shipping companies get daily and weekly reports on water levels from pilot companies in Argentina. This thesis investigates if the data from AIS can be used to make even more accurate and long-term water level predictions for the company. The students have based the prediction model on a rain variable that uses both historical data and future weather predictions. This becomes a way to compare information, which can be useful because predicting too low water levels can as mentioned, lead to big losses for the company. Until now, they have depended on numbers from an external company in the Paraná River. It can be an advantage to have the information internally and compare the results with external ones. As more historical data becomes available in the open data source AIS, one can probably also expect to see more accurate results.
The master’s students have used time-based cross-validation to prepare the prediction models. That means dividing the data into two sets: one training set and one test set. The idea here is that the model continuously looks at more and more historical data in the training set as it becomes available. The available historical data will then be used to predict the coming 12 weeks, which is the test-set (see Figure 14 below). This distribution starts already in 2016, so the first training set contains 4 years of data, and you can see how well the model predicts the water level as new weeks of AIS-reported draft become available.
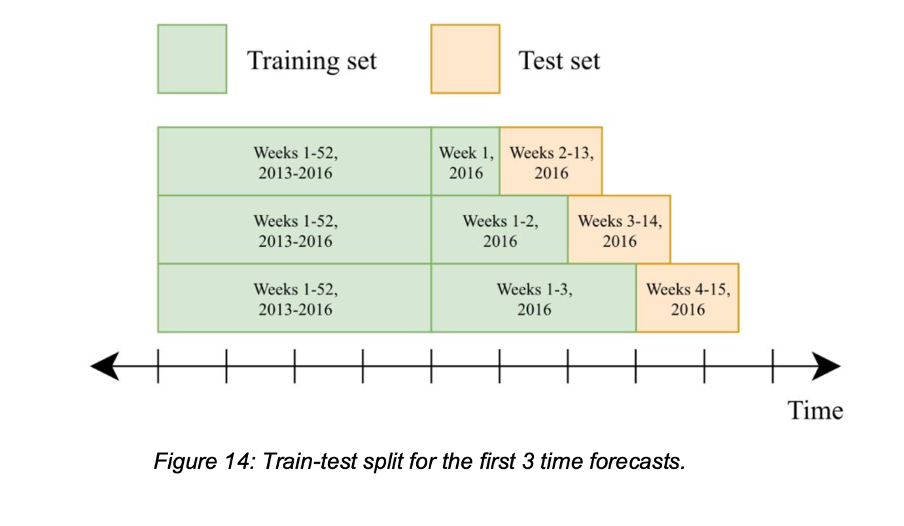
The results indicate that high-frequency AIS-reported draft works better and gives a better basis for price estimation of the cargo than regularly reported water levels, which is what Western Bulk uses now. The model gives estimated water level margins 1-12 weeks ahead and can be used by shipping companies to improve cargo estimation and reduce risk.
The master’s thesis is largely concerned with how climate change creates more uncertainty in the shipping industry. If climate change leads to a percentage increase in the rain from one year to the next, predicting water levels will become more difficult. As we have seen, this can be a problem for the shipping company, but with new methods, such as those explored here, we can try to solve them.
Connection to Climate Futures
Climate Futures wants to develop new ways to handle climate risk in the shipping industry. By using climate forecasts that exceed a couple of days ahead, one can make informed risk evaluations.
The connection between this master’s thesis and Climate Futures is two-fold. Firstly, climate change will affect production, and thus the volume, of shipping export of agricultural goods, like grain from South America. Secondly, climate change affects the infrastructure that is used for this export – in this case, the water level in one of the main rivers for international ships. This will again affect how much cargo each ship can carry, and consequently also transport costs and the profitability of the companies, like SFI-partner Western Bulk. It is therefore interesting for them to improve the prediction and modelling of precipitation and water levels on a relatively short time scale, what we call sub-seasonal forecasts, or weekly to monthly forecasts.
How will the smart-shipping node continue to work on this topic?
The goal is for this master’s thesis to be further developed into a scientific publication, preferably in cooperation with the Renewable Energy node. This node also has projects in South America, which has an overlapping interest in improving the precipitation forecasts in the area.